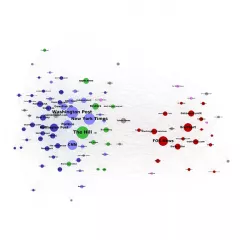
Understanding Media and Information Quality in an Age of Artificial Intelligence, Automation, Algorithms and Machine Learning
This post looks at some of the lessons we’ve learned over the last year in our work as part of the Ethics and Governance of AI Initiative, a collaboration of the Berkman Klein Center and the MIT Media Lab.
Democracy is under stress that would have been unimaginable a decade or two ago. The victory of Donald Trump in the U.S. presidential campaign and the successful “Leave” campaign in the UK Brexit referendum led the Oxford English Dictionary to select “post-truth” as word of the year for 2016. Since then, anti-elite populism across the North Atlantic has continued to gain victories, chief among them the 2018 victory of the Five Star Movement in Italy. As governments, foundations, academics, political activists, journalists, and civil society in democratic societies struggled to understand the overwhelming political results alongside the apparent information disorder, most observers’ eyes fell on technology. Social media, bots, hyper-targeted behavioral marketing — all have been seen as culprits or at least catalysts in public conversations. Technology has altered the foundations of news and media, and as trust in media continues to decline, artificial intelligence, machine learning, and algorithms have come to play a critical role not only as threats to the integrity and quality of media, but also as a source of potential solutions.
The core threats to information quality associated with AI include:
- Algorithmic curation. Most commonly known as the “filter bubble” concern, algorithms designed by platforms to keep users engaged produce ever-more refined rabbit holes down which users can go in a dynamic of reinforcement learning that leads them to ever-more extreme versions of their beliefs and opinions.
- Bots. Improvements in automation allow bots to become ever-more-effective simulations of human participants, thereby permitting propagandists to mount large-scale influence campaigns on social media by simulating larger and harder-to-detect armies of automated accounts.
- Fake reports and videos. Improving automated news reporting and manipulation of video and audio may enable the creation of seemingly authentic videos of political actors that will irrevocably harm their reputations and become high-powered vectors for false reporting.
- Targeted behavioral marketing powered by algorithms and machine-learning. Here, the concern is that the vast amounts of individually-identifiable data about users will allow ever-improving algorithms to refine the stream of content that individuals receive so as to manipulate their political opinions and behaviors.
Our approach to this set of issues is through the analysis of very large datasets, covering millions of stories, tweets, and Facebook shares, across diverse platforms reflecting national presidential politics in America from April 2015 to the present. The results of our work suggest that emphasizing the particular and technological, rather than the interaction of technology, institutions, and culture in a particular historical context, may lead to systematic overstating of the importance of technology, both positive and negative. Many studies do not attempt to address the impact of AI and other technologically-mediated phenomena that they identify and measure, but rather are focused on identifying and describing observable practices. In our own work, we have repeatedly found bots, Russian influence campaigns, and “fake news” of the nihilistic political clickbait variety; in all these contexts, we have found these technologically-mediated phenomena to be background noise, rather than a major driver of the observed patterns of political communication. Excessive attention to these technology-centric phenomena risks masking the real political-cultural dynamics that have confounded American political communication.
Studying media ecosystems
We study propaganda and disinformation in political communication through the lens of media ecosystems. The springboard for our research is a tool built in a decade-long collaboration between our Berkman Klein Center team and our colleagues at MIT Media Lab’s Center for Civic Media: Media Cloud. Media Cloud enables us to discover media network structures that form around specific topics and to shed light on media frames, attention, and influence. Our early work centered on discrete legislative and regulatory controversies, such as net neutrality and the SOPA-PIPA bills. As election year approached in 2016 we turned our attention to the presidential race.
Initially, we analyzed the 18 months leading up to the November 8 vote. The Columbia Journalism Review published our preliminary findings in February 2017, and we followed with a more complete analysis of pre-election media in August of last year. Since then we have expanded our research to include analysis of the election’s aftermath, and have combined the election-period study with a review of the first year of the Trump presidency into a book coming out with Oxford University Press in September 2018, entitled Network Propaganda: Manipulation, Disinformation, and Radicalization in American Politics. With the support of the Ethics and Governance of Artificial Intelligence Fund, we focused attention on the impact of artificial intelligence and automation on political public media. This work is motivated by the need to better understand the character, scope, and origins of the issue as an essential element in crafting corrective measures. Here we summarize our preliminary conclusions as well as the work of a growing number of researchers investing in this space.
Disinformation problems are fundamentally political and social — not technological
Our research leads us to conclude that political and social factors, not technology, are at the root of current media and information quality problems in American political communication. We analyze the linking, tweeting, Facebook sharing, and text of four million stories related to presidential politics published over a period of nearly three years before and after the 2016 presidential election. Relying on this data, we mapped out media source networks in open web media (online news, blogs, etc.) and on Twitter to learn how media sources clumped together based on similar cross-media linking and sharing patterns. This revealed a startling finding: media sources are, as many have observed, deeply polarized; but they are aligned in a distinctly asymmetric pattern. There is, as it turns out, not a simple left-right division in American political media. Instead, the divide is between the right and the rest of the political media spectrum. Media sources ranging from those with editorial voices that lean towards the center-right, such as the Wall Street Journal, all the way to distinctly left voices such as Mother Jones or the Daily Kos, comprise a single interconnected media ecosystem. In this portion of the media spectrum, attention is normally distributed around a peak focus on mainstream professional journalism outlets and journalistic values and mainstream professional journalism continue to exercise significant constraint on the survivability and spread of falsehoods. By contrast, there is a large gap between media sources in this broad center to center-left to left cluster and media sources on the right. Moreover, attention on the right increases as one moves further from the center, and peaks around media sites that are exclusively right-wing.
Network map based on Twitter media sharing from January 22, 2017, to January 21, 2018. Nodes are sized by number of Twitter shares.
When we took a closer look at the substance and practices of journalism from the center of the spectrum to its poles, we found major differences in behavior. Media sources on the left and right extremes of the spectrum are prone to sacrificing accuracy in favor of partisan messaging, making them also prone to the spread of disinformation. However, the accountability and fact-checking mechanisms in the media structures on the center and center-left provide a barrier against further propagation and a check on shoddy reporting from the left. Media outlets that strive for objectivity and balance are less susceptible to spreading disinformation.
The leading media in the right-wing cluster follow a distinctly different strategy, and repeatedly amplify and accredit, rather than dampen or correct, bias-confirming falsehoods and misleading framings of facts. We find that insular conservative media lack the will or capacity to adhere to objectivity and accuracy in their reporting. Infowars, Gateway Pundit, and conservative political clickbait are a problem, but a bigger problem is when Drudge Report, Breitbart, and Fox News — conservative media sources with much larger audiences — amplify conspiratorial and deceptive stories. This creates a significant gap in information quality for readers of conservative-leaning sources of news relative to those who consume media sources outside the right-wing ecosystem, including left-wing sites.
Algorithmic curation and filter bubbles
It is important to understand that if filter bubbles or algorithmic curation were the primary reason for polarization, we would expect to see symmetric patterns of attention clustering by populations of audiences and publisher — all of whom are at the same technological frontier. The stark asymmetry between the right and the rest strongly suggests that differences in political culture and, we argue in our book, institutionally-driven media history of right-wing media, particularly talk radio and Fox News, drove the right into a media dynamic we call the propaganda feedback loop. This occurred before major Internet sites developed; Internet sites adapted to that already-operative media dynamic, rather than causing it.
Indeed, when we compare the pre-election coverage and the post-election coverage, attention on the left has shifted more to the center, while attention on the right has remained as right-oriented. Fox News has become more prominent online relative to Breitbart, but at the price of becoming more purely focused on right-oriented content than it was during the election. Fox online has lost hyperlinks from the center-right and center even more than from the center-left and left, and gained links from the right. This pattern is not the simple product of algorithmic filter bubbles. Compared to influence metrics based on social media attention, weblinks are more insulated from platform-imposed algorithms as they reflect the judgment and linking decisions of web authors, rather than attention and sharing patterns of audiences. Nonetheless, the asymmetric polarization appears by this measure too, and it is by this measure that Fox itself has moved to the right since the 2016 election.
Bots
Over the course of the year we have developed our own versions of several of the most widely-used bot-detection algorithms. We reran all of our analyses after removing accounts that were identified as “bots” by various methods. Although we do in fact identify a good number of accounts that would be considered “bots” by many of these definitions, the overall architecture of communication we observe remains unchanged whether we include these accounts or not. Without stronger evidence that particular major communications dynamics have been swayed by bots, our macro-level observations leave us skeptical as to the importance of bots. Moreover, using micro-scale case studies, we observe bots as background noise rather than as impactful interventions.
Functional and structural differences
Today’s media ecosystems are of course inseparable from technology, but if technology was at the core of the disinformation problem, we would expect to see as much disinformation on one side of the spectrum as the other. And yet, that’s not the case. The asymmetry we observe in the structure and practices of media across the U.S. political spectrum is evidence that the dynamics and problems in modern day media cannot be explained by technology alone.
Our caution should not be taken as clear evidence that algorithms are unimportant. There is little doubt in our minds that algorithms and machine learning impact media manipulation and disinformation. In large-scale digital media platforms, algorithms serve a curatorial role and act as gatekeepers, and are subject to gaming and manipulation in ways that human editors are not. In our own research, we have seen, for example, that Twitter is littered with disinformation and false accounts, some of which are bots and others sockpuppets. Moreover, we observe that the spread of disinformation is worse on Facebook than on Twitter, and on Twitter than on the open web. Moreover, we observed that, as Facebook was making its algorithm changes over the year, certain of the worst offenders on both the left and the right lost prominence and attention, although new offenders quickly took their place. In our current work we continue to monitor the differences across platforms and to find measures by which to assess the effectiveness of algorithm changes on Facebook or Twitter on the spread of disinformation and misinformation, and, perhaps most importantly, to assess the impact of these changes on the media ecosystem as a whole.
Diagnoses, conclusions, and interventions should be based on systemic observations of impact, rather than individual observations of “bad behavior”
The media manipulation problem and the public attention afforded it have created an environment of urgency. Insights and research are key to mitigating some of this pressure, but the temptation to leap to assumptions of impact from any observable phenomenon is hard to resist. We are naturally drawn to the novel: trolls, clickbait factories, social media algorithms, targeted advertising, psychographic profiling, and Russian interference captivate the imagination and compel question and inquiry. Identifying instances of such interventions is technically challenging, and when we see hard work bear fruit it is tempting to attribute high importance to these observations. Nonetheless, without a baseline against which to assess the impact of one or several of these phenomena, it is impossible to justify assigning them the importance that they currently receive, or to design interventions that properly address the actually important interventions rather than those that are observable and salient.
Putting these novelties into perspective is hampered by our ability to fully and accurately measure media consumption. Audiences reached by misleading and false articles and media sources is measured in the millions. As prolific as this sounds, as described previously this ultimately is a small portion of content and exposure. A second point of uncertainty relates to impact and persuasion — there is scant evidence that false media stories have any impact in changing behavior.
A prime example is Russian efforts to influence election-related discourse. Although information around the number of accounts, posts, and impressions seems to be updated regularly, current numbers draw into question the true significance of their reach. In October 2017, Facebook revealed that approximately 80,000 pieces of content published by the now infamous Russian-operated Internet Research Agency were introduced to 29 million people between January 2015 and August 2017. Their subsequent likes and shares increased the reach of the posts to 126 million Facebook users. This is indeed a big scary number. But what we don’t know is how much user attention this content commanded among the many billions of stories and posts that comprised the news feeds of U.S. voters, or if it changed any minds or influenced voter behavior. For example, using one of the tools we developed for this project, we can track the appearance of a given string across Facebook public facing pages, Twitter, Reddit, Instagram, and the first few posts in 4chan discussions on a timeline down to the minute or second (depending on the platform). Using that utility, we tracked a particular story with strong indications of Russian influence (about John Podesta participating in satanic rituals), and compared all accounts to both the congressionally-released list of Russian Twitter accounts and an additional list of tens of thousands of accounts that are highly likely to be Russian bots or sockpuppets. While we did observe that 5–15% of the accounts were of such provenance at any given minute, the timing and pattern of their participation was unremarkable — a background presence of more-or-less stable existence, rather than a strategic intervention in either timing or in direction, and they therefore did not seem to play an important role in increasing the salience of the story in total or by tweeting it specifically at the major actors who were central in propagating the lie — Wikileaks, Infowars, or, ultimately, Sean Hannity.
It is critical not to confound the observation of a novel phenomenon with its actual impact in the world; newness is no proxy for impact. Indeed the discovery of such phenomena is important, but the messaging around the discovery should not be conflated with evidence that the discovered behavior in fact had impact.
The rapid advancement of technologies that digitally manipulate sound, images, and videos is a source of growing anxiety. It is too soon to predict whether synthetic media will exacerbate media disorder and epistemic crisis, but if fabricated media are shared, consumed, and interpreted in ways similar to the current versions of deceptive media, it will be spread or contained by the same influential social, political, and media entities. The fantastical nature of the claims that were widely believed by significant numbers of voters — nearly half of Trump voters gave some credence to rumors that someone associated with the Clinton campaign was running a pedophilia ring — suggests that social identity, much more than any technical indicia of truth, are the foundation for beliefs and attitudes about political matters.
Our work greatly benefits by consciously focusing on the disinformation forest, rather than on its individual trees. With an eye toward the long-term dynamics between institutions, culture, and technology, we look at algorithms and technology as a relatively new element integrated into the dynamics of broader media systems while keeping grasp of many more traditional vectors and structures. We look across media and across platforms, considering the enduring influence of radio and cable alongside broadcast television and social media platforms. Indeed, a combination of open web media publication and social media network mapping greatly enhances our understanding of the likely impact of bots, sockpuppets, political clickbait, foreign intervention, and microtargeting. Although prolific in absolute terms, the role of these modern vectors of disinformation plays an important but modest role in the overall media ecosystem.
It doesn’t look like AI is coming to the rescue anytime soon, if ever
There are a number of ways in which AI may help us to address information quality issues, including:
- Automated detection and flagging of false stories; algorithmic tools to point readers to corrections and fact-checking.
- Algorithmic tools to detect and defend against coordinated attacks by propagandists.
- Computational aids to “nudge” of readers and users to expose themselves to media and reporting outside of their echo chambers, thus opening their eyes to a broader range of viewpoints.
Despite the strong advances in the field, there is little indication at present that these tools will be able to serve as independent arbiters of truth, salience, or value. Repeatedly in our work, we have seen that stories built around a kernel of true facts, framed and interpreted in materially misleading ways are more important than stories made of clear falsifiable claims. Moreover, the meaning of stories often emerges from the network of stories and frames within which they are located. Stories and their interpretation — and misinterpretation — draw upon and act through existing social and political structures and narratives. As seen in existing applications, AI is effective at channeling and amplifying sentiments and interests of affinity groups, and helping people in ways that they want to be helped, but no present efforts appear to be able to diagnose the level of subtlety involved in the most important present disinformation campaigns we have been studying.
Systemic responses are required to address systemic issues
So what do we do about all of this? There is no silver bullet, but the best thing we can do is to continue to work toward systemic responses to the systemic problems undermining today’s media ecosystem and information quality. We must continue to focus attention on rebuilding and strengthening the political and civic institutions that undergird a well functioning democracy. The insights described here draw primarily on research conducted in the United States, but we need ecosystem-based analyses of media systems in more countries to understand how the vulnerabilities, resilience, and dynamics differ across different political and cultural contexts.
Strengthening media accountability mechanisms is vital, and tamping down disinformation and media manipulation is an integral part of the everyday hard work of democracy and governance. But given the perils of placing constraints on political speech, taking a careful and cautious approach to regulation is essential. It is important to be similarly circumspect in what we require of social media companies in addressing disinformation.
Last but far from least, there is still much work to be done in understanding the nature, reach, and impact of disinformation, as well as monitoring and evaluating targeted efforts to inoculate readers and inhibit the spread of disinformation. An area of particular concern is the increasing concentration of research capability and political outreach capability in a few private hands. We must continue efforts to bolster public interest research initiatives and develop and implement structures that allow researchers access to key sources of data with mechanisms in place to ensure robust and ethical research standards.
The development, application, and capabilities of AI-based systems are evolving rapidly, leaving largely unanswered a broad range of important short- and long-term questions related to the social impact, governance, and ethical implementations of these technologies and practices. Over the past year, the Berkman Klein Center and the MIT Media Lab, as anchor institutions of the Ethics and Governance of Artificial Intelligence Fund, have initiated projects in areas such as social and criminal justice, media and information quality, and global governance and inclusion, in order to provide guidance to decision-makers in the private and public sectors, and to engage in impact-oriented pilot projects to bolster the use of AI for the public good, while also building an institutional knowledge base on the ethics and governance of AI, fostering human capacity, and strengthening interfaces with industry and policy-makers. Over this initial year, we have learned a lot about the challenges and opportunities for impact. This snapshot provides a brief look at some of those lessons and how they inform our work going forward.