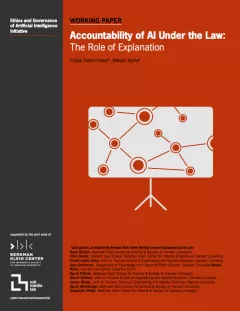
Accountability of AI Under the Law: The Role of Explanation
by Finale Doshi-Velez and Mason Kortz
for the Berkman Klein Center Working Group on Explanation and the Law:
Chris Bavitz, Harvard Law School; Berkman Klein Center for Internet & Society at Harvard University
Ryan Budish, Berkman Klein Center for Internet & Society at Harvard University
Finale Doshi-Velez, John A. Paulson School of Engineering and Applied Sciences, Harvard University
Sam Gershman, Department of Psychology and Center for Brain Science, Harvard University
Mason Kortz, Harvard Law School Cyberlaw Clinic
David O'Brien, Berkman Klein Center for Internet & Society at Harvard University
Stuart Shieber, John A. Paulson School of Engineering and Applied Sciences, Harvard University
James Waldo, John A. Paulson School of Engineering and Applied Sciences, Harvard University
David Weinberger, Berkman Klein Center for Internet & Society at Harvard University
Alexandra Wood, Berkman Klein Center for Internet & Society at Harvard University
Abstract
The ubiquity of systems using artificial intelligence or "AI" has brought increasing attention to how those systems should be regulated. The choice of how to regulate AI systems will require care. AI systems have the potential to synthesize large amounts of data, allowing for greater levels of personalization and precision than ever before|applications range from clinical decision support to autonomous driving and predictive policing. That said, common sense reasoning [McCarthy, 1960] remains one of the holy grails of AI, and there exist legitimate concerns about the intentional and unintentional negative consequences of AI systems [Bostrom, 2003, Amodei et al., 2016, Sculley et al., 2014].
There are many ways to hold AI systems accountable. In this work, we focus on one: explanation. Questions about a legal right to explanation from AI systems was recently debated in the EU General Data Protection Regulation [Goodman and Flaxman, 2016, Wachter et al., 2017], and thus thinking carefully about when and how explanation from AI systems might improve accountability is timely. Good choices about when to demand explanation can help prevent negative consequences from AI systems, while poor choices may not only fail to hold AI systems accountable but also hamper the development of much-needed beneficial AI systems.
Below, we briefly review current societal, moral, and legal norms around explanation, and then focus on the different contexts under which explanation is currently required under the law. We find that there exists great variation around when explanation is demanded, but there also exists important consistencies: when demanding explanation from humans, what we typically want to know is how and whether certain input factors affected the final decision or outcome.
These consistencies allow us to list the technical considerations that must be considered if we desired AI systems that could provide kinds of explanations that are currently required of humans under the law. Contrary to popular wisdom of AI systems as indecipherable black boxes, we find that this level of explanation should often be technically feasible but may sometimes be practically onerous|there are certain aspects of explanation that may be simple for humans to provide but challenging for AI systems, and vice versa. As an interdisciplinary team of legal scholars, computer scientists, and cognitive scientists, we recommend that for the present, AI systems can and should be held to a similar standard of explanation as humans currently are; in the future we may wish to hold an AI to a different standard.
The authors have invited researchers, technologists, and policy makers to engage with the ideas outlined in the paper by emailing [email protected] and [email protected]. For questions and comments related to broader AI themes and/or related activities of the Ethics and Governance of Artificial Intelligence Initiative, please email [email protected].